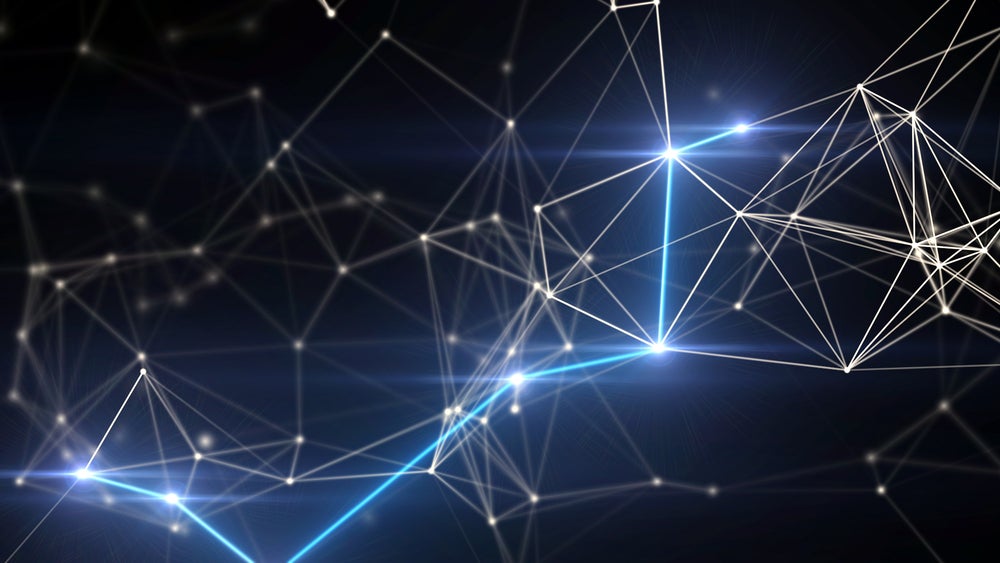
Why is it important for a data science job candidate to show, and not just tell? Because finding jobs as a data scientist can be difficult without relevant work experience and it’s very difficult to argue against evidence.
Employers are looking for people who can do, rather than those who simply say they can. And because the candidate who provides living examples of their talent immediately places themselves in the leading pack, well ahead of the peloton, in the race for well-paid data science jobs with employers of choice.
Dr Neil Fraser from the College of Science and Engineering at James Cook University says all too often, CVs are read by robots looking for keywords that use an algorithm to automatically create a short list of potential candidates.
“The job-seeking data scientist needs to outsmart the machine,” he says.
“This is where keywords, industry-specific terms and particularly portfolios of work become effective in finding those well-paid jobs.”
“To outsmart the machine you will need to have thought through a strategy for securing that role, which would include a portfolio that includes the keywords, with links to your online projects, all with relevant industry-specific terms. A good source of data science keywords and definitions can be found on Data Science Central, a handy reference for writing up your portfolio and updating a resume.”
Once keywords are taken care of, it’s time to build a killer portfolio.
Building a data science portfolio
What does a brilliant portfolio look like? What exactly is it that employers are looking for? How does a graduate with little real-world job experience prove they’ve got what it takes?
Of course, it depends on the employer and the role they’re looking to fill, but the one trait that every employer is seeking is initiative. If you can prove that you have taken on and completed several projects, that you have earned and utilised relevant knowledge and you are proud enough of the work you have done to have made it public - that’s an irresistible combination for any employer.
Topic areas in data science are many and varied. The knowledge areas overlap with fields such as computer science, statistics, machine learning, data mining, operations research and business intelligence. Any of these areas - and more - can be included in your portfolio, Dr Fraser says.
In fact, the clearer the intent of the project and the more defined its audience, the greater its chances of demonstrating the type of deep knowledge and application that employers are likely looking for.
“The project needs to be clear about which audience it is aiming at and the discipline focus,” Dr Fraser says. “It is very important to explore new datasets and avoid well-known ones, as these are too often done by every would-be data scientist and therefore appear less authentic in portfolios. An example [of one to avoid] would be the Iris Dataset. Also, as obvious as it might seem, target a project at the industry you are interested in working within.”
Where should the portfolio be found?
Some young graduates publish their research papers on academic websites and others publish detailed blogs. Some offer their data on major online platforms such as Kaggle and others share their code on Github.
And some build their own website where all of their work is openly available while others share their expertise in a particular area, actively answering online queries on popular websites and discussion boards frequented by industry professionals. After all, data scientists within organisations turn to the platforms they know best in order to help solve their own problems. If you become known as a solver of problems, that’s a very good start in the employment world.
All of the above are excellent choices and each will put the graduate a good distance ahead of the candidate who simply writes an impressive CV.
What is important, of course, is the accessibility of the work. If it is published on a platform that is only open to members, that is password protected or that requires pay per view, it will not work as a tool to engage potential employers.
“The candidate’s portfolio needs to be accessible online through a platform such as Github, Anaconda or Tableau Public, as evidence of authenticity,” Dr Fraser advises.
“The code or workbook repository should be well commented, clean and tidy, in an easily accessible format and with a useful readme file to explain the project.”
“If an employer or talent scout needs to log in to get access to your work then it will not often be viewed, so be prepared to share your work openly.”
“In summary, a portfolio must include data science keywords, with links to your online portfolios and all with relevant, industry-specific terms. It also needs to be clear and focused by discipline and industry sector, to avoid any ambiguity for the audience around what is being demonstrated.”
Learn more about studying data science online at postgraduate level, enquire now by calling our Enrolment team on 1300 535 919.
-
Previous post
Finding a balance between technical and soft skills in data science -
Next post
Corporate strategy: Essential for business growth
Find out more about JCU’s online Master of Data Science.
Get in touch with our Enrolment team on 1300 535 919