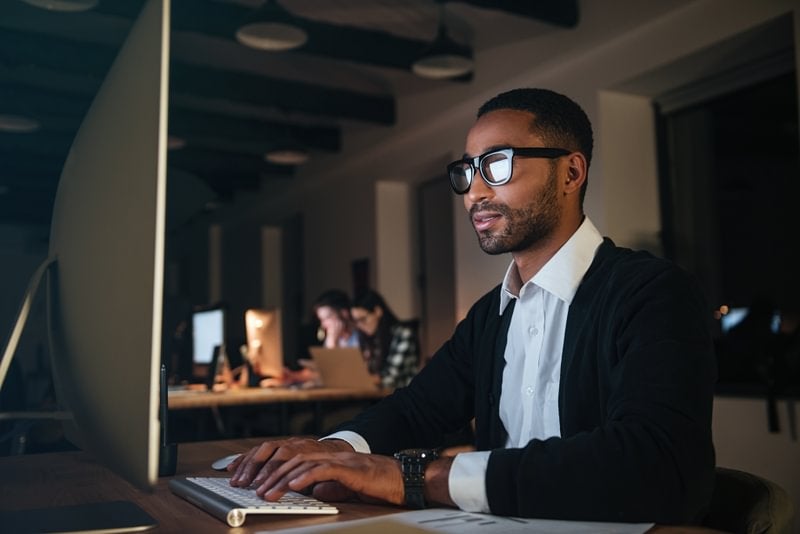
Data scientists in Australia are predicting the future. Data science skills such as predictive modelling use data mining and machine learning to help businesses forecast everything from customer decision-making to corporate profits, according to Oracle NetSuite.
According to the Department of Industry, Science and Resources, Australian data scientists are meeting challenges and helping boost productivity and supply innovative solutions, in major industries such as health, agriculture and mining.
Individuals who gain cutting-edge skills can become part of the vanguard of professionals shaping our global future through a rewarding data science career. One way to learn the data science skills required by employers is to study an advanced degree, such as a Master of Data Science.
There are a wide range of expertise required of today’s data scientists. These skills are divided into two major categories: technical skills and soft skills.
Data science technical skills
In the field of data science, technical skills consist of specialised expertise – such as a working knowledge of programming languages and other technical concepts – for performing detailed technical operations and applying them to real-world issues.
1. Mathematics
The foundational skill of data science is mathematics. An aptitude for (and an enjoyment of) maths is instrumental to developing the other technical skills data scientists need. The logic and theories in maths lend themselves directly to the development of data models and algorithms that data scientists apply to solve business problems. This practical application makes mathematical knowledge and ability a key skill required by today’s employers.
2. Statistics
Mathematical skills are necessary for developing expertise in another fundamental data science concept – statistics. Key statistical concepts include:
- Appropriate sampling techniques: particularly crucial when applying experimental conditions and/or evaluating the provenance of the data.
- Data distributions: the shape of the data for the most accurate analyses.
- Central tendency: finding the centre of the distribution.
- Dispersion: how much the data varies.
A background in statistics guides the data scientist in determining which statistical test is appropriate for the data and for the business problem at hand.
3. Programming languages
There are a number of programming languages that are essential for data scientists.
Python is one of the top data science programming languages. Many data scientists attest to Python’s ease of use and its flexibility. Python is often used for machine learning as well as for large-scale web applications.
On the other hand, R, a programming language for statistical computing widely used by researchers to analyse and graph data, is a preferred language for many disciplines, including academia, for exploring and experimenting with data. Learning both Python and R is strongly recommended.
SQL is also desirable for querying from relational databases. The field still considers older programming languages such as C/C++ useful as well.
4. Data engineering
Much of a data scientist’s time is spent on sourcing and preparing data – certainly as much as it is on developing algorithms and models. Thus, a vital data science technical skill is familiarity with the extract, transform and load (ETL) process:
- Extract data from a source.
- Transform data by formatting it for the destination database/storage.
- Load data by transferring it into the system used for analysis.
The ability to wrangle data is particularly crucial when dealing with unstructured, undefined data sets (such as those extracted from social media or blogs).
5. Machine learning
Machine learning is a subset of artificial intelligence. Software applications in this arena teach a machine how to detect patterns and themes in data without a specific set of instructions. Machine learning is a basic data science skill – it assists the data scientist in analysing huge volumes of data efficiently because it allows for real-time data processing.
6. Data visualisation/storytelling
Displaying complex information is a critical data science concept. Well-designed charts and graphs can convey at a glance what might otherwise take several paragraphs of summative text to describe.
Design thinking (the way we reason when thinking about design problems) is a technical skill in this context – data visualisations are key in assisting non-technical decision-makers and stakeholders in comprehending the implications of data and results. Being an effective data scientist involves effectively telling the complete story of the data, from its origins to its ultimate meaning.
One data science skill required for data visualisation is proficiency in software programs such as Tableau, an interactive program used for business intelligence. Expertise in data-driven storytelling tools, such as Canva, is also a valuable skill.
Data science soft skills
Non-technical skills, often referred to as soft skills, are interpersonal abilities that employees apply in the workplace, such as communication, teamwork, leadership and decision-making. The data science soft skills that professionals in the field need are similar to those in other industries but applied in specific ways.
7. Critical thinking
A hallmark of any scientist is the ability to remain objective when facing a problem, letting evidence and theory guide solutions. This is critical thinking at work – the objectivity it affords permits data scientists to find ways to minimise bias from the beginning of a project (data sources) to its end (reporting).
Critical thinking underlies the capability to ask the right questions for the most meaningful result. Thinking critically also allows the data scientist to separate relevant information from noise when analysing data.
8. Communication
As in most disciplines, communicating is key to succeeding as a data scientist. Communication occurs at all levels – data scientists must be able to accurately convey their needs and objectives to:
- Others in their team (such as developers)
- Other units (such as marketing)
Data scientists must be proficient in accurately communicating results to key decision-makers and stakeholders so that they can make informed choices about business decisions and strategies.
9. Business acumen
A data scientist should be able to adopt the company’s culture and mission as well as its technical processes.
Because data drives business decisions, data scientists should also understand the importance of the business context of data science. This context provides the framework for data sources, models, algorithms and interpreting results. A data scientist with business acumen will be able to determine the most effective means for leveraging data for a competitive market edge.
In essence, data scientists are not just analysts – they’re business leaders.
10. Commitment to learning and data science education
Common to technical and non-technical data science skills is intellectual persistence, the drive to learn new competencies and develop existing ones. When a data scientist has earned a master’s degree or PhD, it suggests that they have the persistence and drive inherent to success in the field. A data science degree program can offer students essential skills in SAS, Python, R, Tableau and more.
Learn the data science skills required for a rewarding future
Although a career as a data scientist can certainly be financially rewarding, another benefit of the role is its intellectual stimulation. When your career marries technical skills with business savvy, it will rarely be routine.
JCU’s 100% online Master of Data Science offers the opportunity to develop the technical and soft skills necessary to succeed as a data scientist while fast-tracking your career with flexible study that’s customisable to fit your schedule. Discover how you can become part of data science’s bright future.
-
Previous post
How data science and IoT are shaping the future -
Next post
8 effective communication strategies for nurses
Find out more about JCU’s online Master of Data Science.
Get in touch with our Enrolment team on 1300 535 919