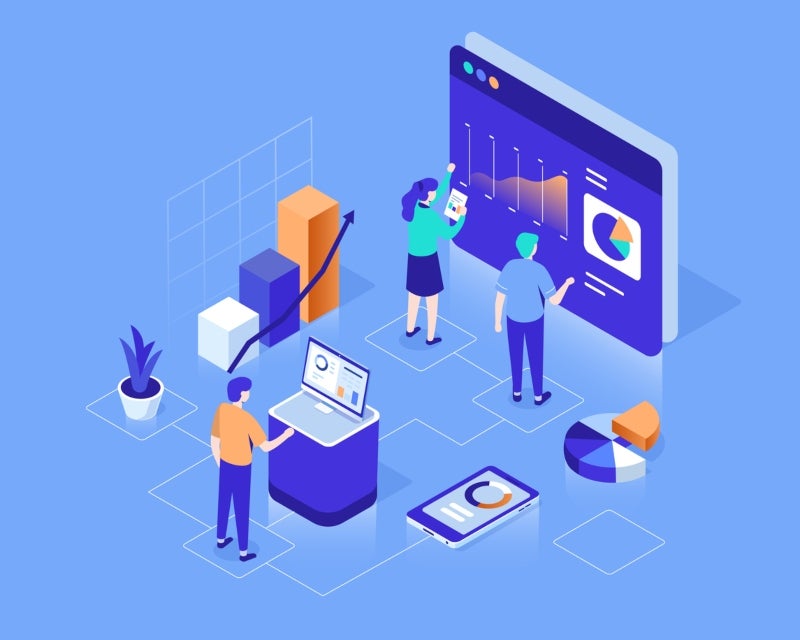
The business world leverages data science for a wide variety of purposes. Between finance, retail, manufacturing, and other industries, the number of ways that businesses can leverage data science is huge, and growing; however, all businesses ultimately use data science for the same reason—to solve problems. Possessing both technical and practical skills, business-focused data scientists understand how to identify which business-relevant problems can best be solved by their particular abilities.
What is a data science problem? A supply chain example
Data scientists don’t solve “analytics problems”, they solve problems that can be solved by analytics. For instance, supply chain efficiency issues are often described as data science problems. Savi, a logistics firm, has even referred to data scientists as the “superheroes of the supply chain”; food companies, militaries, and high tech manufacturing firms are just a few examples of industries that have leveraged data science to improve supply chain efficiency.
Corporations have long recognised an efficient supply chain as being an important element of cost control. Guaranteeing supply chain efficiency requires the ability to account for the various factors that can affect the speed at which products move through a supply chain; because these factors can be described as data, data scientists are able to use them to create models capable of accurately forecasting future scenarios. With modern supply chains becoming ever-more complex, businesses have come to increasingly rely on data scientists for this task. This is particularly relevant when blockchain is applied to manage the provenance of highly valued products like precious gemstones.
The five key data science problems
The particular approach a data scientist must use to solve a business problem varies depending on the needs of their business. Data science is both a science and an art, and the process of solving business challenges relies heavily on the use of creative problem-solving skills.
Below are a few of the ways that data scientists use their skills to solve business problems:
Innovation: Replacing old solutions with new ones
Many of the problems which data scientists address have existed for a long time, and prior to the rise of data science, were managed by other techniques—data scientists often contribute to their company by developing new ways to solve these problems. For instance, through the use of “big data” analytics, data scientists have been able to create consumer demand forecasts which surpass the accuracy provided by previous approaches.
The book/film “Moneyball”—which describes how data science was used to replace traditional approaches to player recruitment in baseball—is one of the best-known examples of a data scientist providing a business with a new solution to an old problem.
Prototyping: Creating new services
The prototyping process is similar to the innovation process, except that the solution being developed is entirely new, rather than replacing an old one. Prototyping is used for the development of both internal services—such as a financial firm using machine learning to monitor for potential compliance breaches—and external, customer-facing services. The use of retail chatbots to guide customer journeys through a sales funnel is a notable example of a service created by prototyping.
Continuous improvement
This is where many entry-level data scientists do most of their work. Continuous improvement is a key principle of modern management practice, and data science is a major driver of it. For data scientists themselves, continuous improvement simply means making an existing data science project work better.
For instance, many business-to-consumer (B2C) firms use data science to target their marketing to specific consumer demographics (segments). This requires data scientists to discover what features distinguish a target audience segment and develop a statistical model capable of recognising those features within a dataset. Because many companies use this tactic, data scientists must continuously improve the models that their firm uses in order to keep them competitive.
Data-value exploration
This type of project is often associated with companies that are just beginning to use data science. Many of these companies have huge amounts of data but have no idea whether any of it is useful. So, it’s up to the data scientist to explore it for potential opportunities. This type of exploratory analysis requires extensive experimentation and relies on the data scientist’s professional judgment and expertise.
As most businesses do not store data in an organised manner, these exploratory projects often require a great deal of data preparation. In such instances, the data scientist must do extensive work to turn disparate data sources into a coherent dataset they can explore in order to find previously unrecognised opportunities.
“Crisis” problem-solving
Countless businesses fail every year because of unidentified or unrecognised problems with their operations. When businesses face unknown problems, data scientists are often able to locate the source of the issue. One common method of doing so is through factor analysis, a form of statistical analysis which allows data scientists to break a process into its constituent parts (factors) in order to determine how much each one is contributing to the problem.
How do data scientists create value for businesses?
Data scientists use a multitude of methods to provide value to a business, but in general, their work can be described according to a basic series of steps.
Step 1: Finding the business case
A data scientist’s workflow begins with research into how they can best provide value to their company. Should they improve its forecasting models? Develop a customer-facing product or service? The answer depends on the data available and the priorities of the business.
At some large enterprises, data scientists function as though their employer is also their primary customer. Data scientists at such firms have a higher degree of independence and work as innovators and prototypes to create or discover new opportunities related to their company’s products and services. In order to maximise the productivity of their data science operations, businesses which use data scientists in this manner may organise their data science departments into semi-independent “Centres of Excellence”.
In other business environments, data scientists will focus on identifying inefficiencies or improving existing projects (continuous improvement). The business case for a data science project will usually be driven by the priorities of the business and its executive leadership; for instance, a data scientist at a B2C firm that prioritises high sales volumes would be expected to focus on projects that will improve sales, such as by improving the firm’s use of marketing analytics.
Step 2: Data collection and engineering
Once a business case has been identified, a data scientist must gather the data necessary for their project. Most data is unstructured and “messy”, and the process of integrating disparate sources of data into a cohesive whole can be a time consuming and technically challenging process; however, a data science project cannot function without this step. The practice of preparing data for use in data science projects is called data engineering and is recognised as its own sub-discipline of data science.
Many marketing-related projects emphasise this step, as they rely on customer data that comes from a variety of different sources. Moreover, they rely on the ability to combine these existing data with new customer data in order to automate functions such as product suggestions. This requires ensuring that all data is relevant and compatible so that the performance of the data scientist’s models is not negatively affected by missing or low-quality data.
Step 3: Data modelling
After collecting the data necessary for their project, the data scientist must turn it into something useful. This involves using statistical models to analyse the data for useful information (i.e., what most people think of as “doing data science”), and then simplifying that information (e.g., through data visualisation) to make it useful to non-data scientists, such as executives.
The business world places a premium on the modelling process. Data scientists aren’t hired to perform one-off ad-hoc analyses, they’re expected to create “robust” models that can be reused for multiple analytics jobs related to a specific function. This is especially true for models that need to be deployed into “production” environments; for example, if a data scientist develops a product recommendation engine, they need to ensure that their model will produce accurate results for everything it’s used for and that it will continue to do so for the foreseeable future.
Step 4: Operations/production
Integrating a data science project into a business’s ongoing operations is the final step of a business data science workflow. The production process is what makes data science projects into data science products, and is the key element through which data scientists provide businesses with a positive return on their investments. While ad-hoc analytics have a role to play in the business world, the data scientist’s ability to create durable models which provide lasting value is what has driven the corporate world’s embrace of the discipline.
Moving a project into production usually requires it to be integrated into a larger tech stack, a process which requires the skills of other tech experts. For instance, a data science project built to provide a business with real-time operational analytics would require a constant stream of data to be sent from multiple sources, combined into a single dataset, and then sent to the data scientist’s analytics models for processing. This is a complex data management task, and would likely require the expertise of a data architect to do successfully.
Note: This step may be omitted in scenarios where the data scientist is attempting to find the answer to a one-off question, such as when they are attempting to locate the source of a specific problem.
Learn these skills at JCU Online
Data scientists working in business must possess strong problem-solving capabilities and project management skills, as well as the ability to identify strategic opportunities for their employer. Moreover, they must develop the communication abilities necessary to get key decision-makers to buy-in to their work in order to ensure that work is successfully integrated into the business’s decision-making and production processes.
James Cook University’s Online Master of Data Science program teaches all of these skills. The JCU curriculum emphasises the big data and database skills necessary to understand how to complete data science projects at enterprise-scale, and provides students with experience solving real-world business problems. JCU students don’t just learn how to do something, they learn why to do it, and are taught the professional skills necessary to make strategic judgments about how to create real value for their employers.
From data acquisition to algorithm development, JCU’s Master of Data science includes the training necessary to learn how to apply data science in a business context. Students learn from educators with industry experience, and the program’s emphasis on project-driven learning means that students conclude their degree with a portfolio of work that they can show to employers. If you’re interested in working as a business-focused data scientist, JCU Online’s Master of Data Science provides the skills that are necessary to achieve real-world success.
-
Previous post
Can an MBA create data-driven leadership? -
Next post
The power of global thinking in business
Find out more about JCU’s online Master of Data Science.
Get in touch with our Enrolment team on 1300 535 919